In the MyData Matters blog series, MyData members introduce innovative solutions and practical use cases that leverage personal data in line with MyData values.
Since the 1980s, personal data has been managed in essentially the same way. Organisations aggregate customer information in vast data warehouses, with the assumption that more data is always better to have than less data. Funnels are designed to vacuum up all of the data that could possibly be available, and to store and maintain it indefinitely. The reasoning is simple. The process of identifying and reaching potential customers is difficult, and a 0.05% conversion rate from a digital campaign is considered a success. So the larger the reach, the more likely a transaction.
The digital economy is built upon this assumption – an assumption that rarely receives scrutiny, even as technology advances and new approaches are possible.
Today, however, we now see that it is possible to flip the model on its head to one that is more efficient, respects privacy, and engenders trust. In this new model, rather than enterprises hoarding data in order to identify potential transactions, data sources (originators) send relevant data to relevant entities only at the moment when it is needed. That is what we now see in our My Data Simulator. We see this ultimately running as a network of networks, with billions of one-to-one data-sharing relationships running on common data exchange protocols, mutually understood schema, standardised information-sharing agreements with an audit log.
Being an old postal network employee, I tend to see what we are now building towards as a Recorded Delivery Network for (personal) data; or perhaps ‘like Fedex for Data’ for my North American colleagues.
Consider the diagram below in which we attempt to illustrate this point. What we are saying is that in this model, ANY type of entity can exchange ANY type of data with ANY other type of entity, in real time, and with DATA PROVENANCE. That provenance word is key; it means that all data exchanges are logged – what data moved, from where, to where, for what purpose, on what basis. When that level of plumbing is in place then yes, any party can effectively drop data packets into the network, they find their way to the addressee, and only the right recipient has the keys to open the packet.
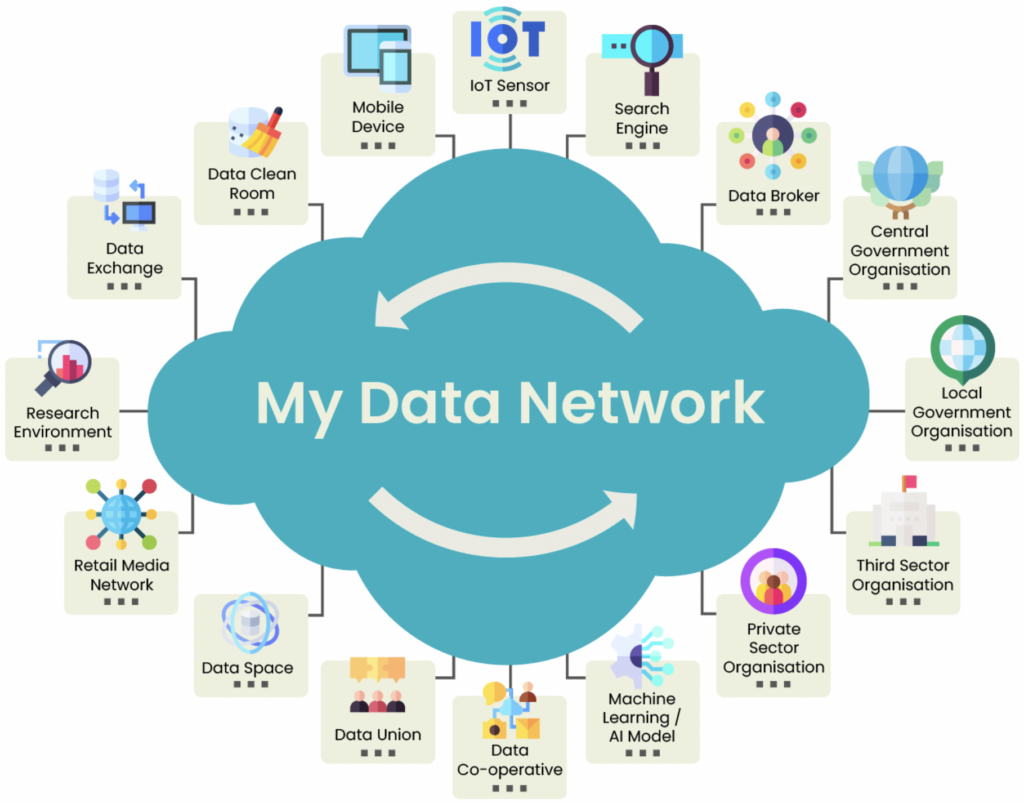
Organisations are bound to the terms of the network-level agreement, and then any specific data-related contract is the same as for any existing data-sharing agreement. Therefore, the ultimate difference in this model is that the individual is a peer node on the network with the advanced capabilities that enable them to act in that way.
In our Simulator we have the above working on a small-scale basis, and with synthetic data; but no barriers to the model scaling – unlike the current model we mention above which is long past its sell-by date.
It would appear to also be the most likely route to gaining the upsides from AI without the downsides. As we all now see only too often, the current model around personal data management often leads to the phrase ‘Garbage in, Garbage Out. Unfortunately, if we take the same approach to ingesting personal data into AI, then we get Garbage In, Poison Out. That is to say, the potential effects of personal data ingested into AI without this provenance layer can be very damaging indeed. In this provenance-enabling network approach, a machine learning model and subsequent AI would have full auditability on the data ingested and driving it.
This is a very similar model to that proposed in the MyData Network of Networks paper; so we look forward to moving this forward with MyData colleagues through this year and beyond.
Author: Iain Henderson is a long-term MyData Member, Architect for Personal Data Solutions at JLINC Labs, and founder of one of the emerging MyData Operators/ data intermediaries – DataPal.